How AI Supports Remote Patient Monitoring
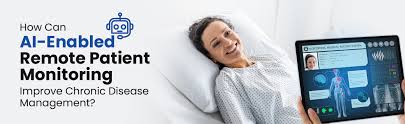
The integration of Artificial Intelligence (AI) into healthcare is fundamentally altering how medical services are delivered, particularly in the realm of remote patient monitoring. Remote patient monitoring (RPM) involves the use of digital technologies to monitor patients’ health outside traditional clinical settings, often from the comfort of their homes. AI enhances RPM by providing advanced data analysis, predictive capabilities, and personalized insights that improve patient care and operational efficiency. This article explores how AI supports remote patient monitoring, detailing its applications, benefits, challenges, and future directions.
The Role of AI in Remote Patient Monitoring
AI plays a pivotal role in remote patient monitoring by leveraging machine learning, data analytics, and advanced algorithms to interpret data collected from various sources. RPM systems typically include wearable devices, sensors, and mobile applications that collect data on vital signs, physical activity, and other health metrics. AI algorithms analyze this data to detect patterns, predict potential health issues, and provide actionable insights for both patients and healthcare providers.
For instance, AI can process data from wearable devices such as smartwatches or glucose monitors to continuously track a patient’s health metrics, including heart rate, blood pressure, and glucose levels. By analyzing these metrics in real-time, AI can identify deviations from normal ranges and alert patients or healthcare providers to potential issues before they escalate into serious conditions.
Current Applications of AI in Remote Patient Monitoring
AI’s applications in remote patient monitoring are diverse and transformative. One prominent application is in chronic disease management. For patients with chronic conditions like diabetes, heart disease, or chronic obstructive pulmonary disease (COPD), AI-powered RPM systems can track daily health metrics and provide real-time feedback. For example, AI can analyze blood glucose levels from a continuous glucose monitor and predict potential hypoglycemic or hyperglycemic events, allowing for timely intervention and adjustment of treatment plans.
In cardiovascular care, AI algorithms can monitor data from wearable ECG monitors to detect arrhythmias or irregular heartbeats. By analyzing patterns in the data, AI can provide early warnings of potential cardiac events, enabling proactive management and reducing the risk of severe complications.
Another significant application is in mental health monitoring. AI-powered tools can analyze data from mobile apps and wearables to assess mood, activity levels, and sleep patterns. This information can be used to monitor patients with mental health conditions such as depression or anxiety, providing insights that can help adjust treatment strategies and enhance overall care.
Benefits of AI in Remote Patient Monitoring
The integration of AI into remote patient monitoring offers numerous benefits. One of the most significant advantages is improved patient engagement and self-management. AI-driven RPM systems provide patients with real-time feedback on their health, empowering them to take an active role in managing their conditions. This increased engagement can lead to better adherence to treatment plans and healthier lifestyle choices.
AI also enhances the efficiency of healthcare delivery. By enabling continuous monitoring and early detection of health issues, AI can reduce the need for frequent in-person visits. This not only lowers healthcare costs but also alleviates the burden on healthcare facilities and providers, allowing them to focus on more complex cases that require in-person attention.
Additionally, AI-driven RPM systems improve the accuracy of health data interpretation. Traditional monitoring methods may rely on sporadic or subjective measurements, while AI can analyze continuous streams of data to provide a more comprehensive and precise understanding of a patient’s health status. This accuracy leads to more informed decision-making and personalized care plans.
Challenges in Implementing AI in Remote Patient Monitoring
Despite its advantages, the implementation of AI in remote patient monitoring faces several challenges. Data privacy and security are major concerns, as the collection and transmission of sensitive health information require robust protection measures. Ensuring compliance with regulations such as the Health Insurance Portability and Accountability Act (HIPAA) and implementing strong encryption and data protection protocols are essential to safeguarding patient information.
Another challenge is the integration of AI systems with existing healthcare infrastructure. Many healthcare providers use disparate systems for managing patient data, and integrating AI-driven RPM tools with these systems can be complex and costly. Ensuring interoperability and seamless data exchange between different platforms is crucial for the effective implementation of AI in RPM.
There is also the issue of data accuracy and reliability. AI algorithms depend on high-quality data to make accurate predictions and recommendations. If the data collected from remote monitoring devices is inaccurate or incomplete, the insights generated by AI may be flawed. Ensuring the reliability and calibration of monitoring devices is essential to maintain the effectiveness of AI-driven RPM systems.
The Future of AI in Remote Patient Monitoring
The future of AI in remote patient monitoring holds great promise, with continued advancements expected in technology and data analytics. Emerging technologies such as advanced sensors, improved wearables, and enhanced AI algorithms will further enhance the capabilities of RPM systems. For example, future developments may include more sophisticated AI models that can analyze a wider range of health metrics and provide even more personalized insights.
The integration of AI with other technologies, such as the Internet of Things (IoT) and blockchain, could also revolutionize RPM. IoT devices can provide additional data points for AI analysis, while blockchain technology could ensure secure and transparent data sharing. These innovations will likely contribute to more accurate, efficient, and secure remote monitoring solutions.
Moreover, AI has the potential to enhance remote patient monitoring by incorporating predictive analytics and preventive care strategies. AI algorithms may increasingly be able to predict potential health issues before they occur, allowing for earlier interventions and reducing the overall burden on healthcare systems.
Conclusion
AI is transforming remote patient monitoring by providing advanced data analysis, real-time feedback, and personalized insights. Its applications in chronic disease management, cardiovascular care, and mental health monitoring are enhancing patient care and improving healthcare efficiency. While challenges such as data privacy, system integration, and data accuracy need to be addressed, the future of AI in RPM is bright. As technology continues to evolve, AI will play an increasingly central role in enabling proactive, personalized, and effective healthcare, ultimately leading to better patient outcomes and a more efficient healthcare system.